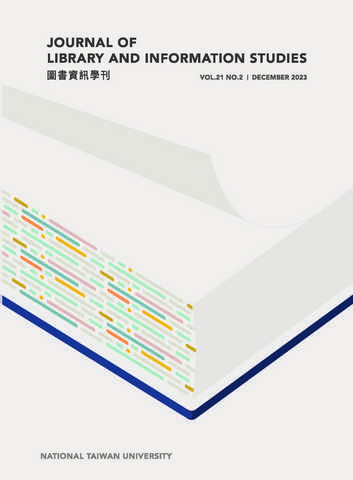 |
本站僅提供期刊文獻檢索。 【月旦知識庫】是否收錄該篇全文,敬請【登入】查詢為準。
最新【購點活動】
|
篇名 |
集成式人工神經網絡模型於分類實務之可行性:以社群媒體之健康消費者資訊分類為例
|
並列篇名 |
Practicability of Ensemble Artificial Neural Network Models for a Classification Task: An Optimal Approach for Reproducing Classification Practices of Health Consumers Generated Text on Social Media |
作者 |
Sukjin You (Sukjin You)、Min Sook Park (Min Sook Park)、Soohyung Joo (Soohyung Joo) |
中文摘要 |
本文運用人工神經網絡(Artificial Neural Network, ANN)模型,再現社群媒體中健康資訊分類實務之準確性。本研究透過Yahoo!Answers健康類別之問答,提取健康資訊術語,並輔以醫學主題詞表(MeSH terms),訓練並比較數種類型的ANN模型和集成式模型的效能。研究顯示,ANN模型分類準確率約90%;其中,深度神經網絡(Deep Neural Network, DNN)與卷積神經網絡(Convolutional Neural Network, CNN)和長短期記憶模型(long short-term memory, LSTM)相比,分類表現更佳。基於分類結果的集成模型不僅優於以基於異質ANN結構的集成模型,也優於單一深度學習模型;本研究也發現問題和最佳答案的組合是最有效的訓練集,並可以建構準確的預測模型。研究結果顯示,ANN模型可有效輔助分類健康消費者以自然語言生成之線上健康資訊。 |
英文摘要 |
This paper reports the classification accuracy of artificial neural network (ANN) models in reproducing health consumers' classification practices in social media. Social media have driven the growth of unstructured text data across domains including health, which motivates researchers to reconsider the epistemological approach to automated classification. This study compared the performance of several types of ANN models and ensemble models based on classification results and the integration of multiple ANN structures. To train these models, two dictionaries were employed: health consumers' terms extracted from questions and answers in the health categories of Yahoo!Answers and MeSH terms. All three types of individual classifiers demonstrated accuracies of around 90%. In particular, the fully connected ANN with two layers produced relatively higher classification performances than a convolutional neural network and long short-term memory. Ensemble models based on classification results outperformed not only the ensemble models based on the integration of heterogeneous ANN structures but also individual deep-learning models. The combination of questions and best answers were found to be most effective as a training dataset to build an accurate prediction model. The findings suggest that ANN models can be an effective assistive tool in classifying online health resources generated by health consumers in natural language. |
起訖頁 |
1-30 |
關鍵詞 |
自動分類、深度學習、人工神經網絡、集成分類模型、知識組織、Automated Classification、Deep Learning、Artificial Neural Network、Ensemble Classification Model、Knowledge Organization |
刊名 |
圖書資訊學刊 |
期數 |
202206 (20:1期) |
出版單位 |
國立臺灣大學圖書資訊學系
|
該期刊-下一篇 |
動態資訊圖像,對小學中年級資源班與普通班之學習影響 |
|